privacy policy
Privacy Policy
Last Updated: 21 Sept 2023
Introduction
Welcome to dimasmukhlas.com. We are committed to protecting your privacy and providing a safe online experience for all our users. This Privacy Policy outlines the information we may collect from you when you visit our website and how we use, disclose, and safeguard that information. You consent to the practices described in this Privacy Policy by accessing or using our website.
Information We Collect
Personal Information
We may collect personal information you voluntarily provide us when you use our website. This may include:
- Your name
- Email address
- Postal address
- Phone number
- Any other information you choose to provide
Non-Personal Information
We may also collect non-personal information automatically when you access our website, such as:
- IP address
- Browser type and version
- Operating system
- Date and time of access
- Pages you visit
- Links you click
How We Use Your Information
We use the information we collect for the following purposes:
-
To Improve Our Website: We may use your information to analyze how our website is used and make improvements to enhance your experience.
-
To Respond to Your Requests: If you contact us with inquiries or requests, we may use your information to respond to you.
-
To Send Emails: If you provide your email address, we may send you periodic updates, newsletters, or other communications related to our website and the more than finance world. You can opt out of these emails at any time.
-
To Protect Our Rights: We may use your information to protect our rights, privacy, safety, or property, or that of others.
Disclosure of Your Information
Without your consent, we do not sell, trade, or otherwise transfer your personal information to third parties. However, we may disclose your information in the following circumstances:
-
Legal Compliance: We may disclose your information when required by law or in response to lawful requests by public authorities.
-
Business Transfers: If we are involved in a merger, acquisition, or sale of all or a portion of our assets, your information may be transferred as part of that transaction.
Security
We take reasonable measures to protect your personal information from unauthorized access, disclosure, alteration, and destruction. However, please be aware that no data transmission over the Internet or method of electronic storage is entirely secure. While we strive to use commercially acceptable means to protect your information, we cannot guarantee security.
Your Choices
You have the following choices regarding your information:
-
Access and Update: You may access and update your personal information by contacting us.
-
Opt-Out: You can opt out of receiving promotional emails from us by following the email instructions or contacting us directly.
Changes to This Privacy Policy
We may update this Privacy Policy to reflect changes to our information practices. We encourage you to review this page periodically for the latest information on our privacy practices.
Contact Us
If you have any questions or concerns about this Privacy Policy or our data practices, please contact us at [email protected].
3 months ago
A reflection of using kanban flow and being minimalist
Recent newsToday is the consecutive day I want to use and be consistent with the Kanban flow! It seems it's perfect to limit my parallel and easily distractedness.
read more3 months, 2 weeks ago
3 months, 2 weeks ago
Podcast Bapak Dimas 2 - pindahan rumah
Recent newsVlog kali ini adalah terkait pindahan rumah!
read more3 months, 2 weeks ago
Podcast Bapak Dimas - Bapaknya Jozio dan Kaziu - ep 1
Recent newsSeperti yang saya cerita kan sebelumnya, berikut adalah catatan pribadi VLOG kita! Bapak Dimas
read more3 months, 2 weeks ago
Happy new year 2024 and thank you 2023!
Recent newsAs the new year starts, I want to revisit what has happened in 2023.
read more3 months, 2 weeks ago
Some notes about python and Zen of Python
Recent newsExplore Python syntax
Python is a flexible programming language used in a wide range of fields, including software development, machine learning, and data analysis. Python is one of the most popular programming languages for data professionals, so getting familiar with its fundamental syntax and semantics will be useful for your future career. In this reading, you will learn about Python’s syntax and semantics, as well as where to find resources to further your learning.
5 months ago
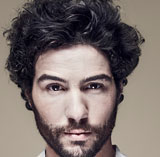
Thanks for stopping by. All the information here is curated from the most inspirational article on the site.