week 3 Finance with Python - access the market data
Posted by: admin 5 months, 4 weeks ago
(Comments)
Week 3: Time Series Analysis and Market Data
Learning Material:
-
Day 1: Time Series Data
- Objective: Learn about time series data and its importance in finance.
- Topics: Time series data, components of time series, and financial applications.
-
Day 2: Working with Time Series Data in Python
- Objective: Learn how to manipulate and analyze time series data in Python.
- Topics: Time series data handling, visualization, and Python libraries like Pandas.
- Code Example: Basic time series analysis in Python.
import pandas as pd import matplotlib.pyplot as plt # Load time series data data = pd.read_csv("time_series_data.csv", parse_dates=["Date"], index_col="Date") # Plot time series plt.figure(figsize=(10, 6)) plt.plot(data.index, data["Price"]) plt.xlabel("Date") plt.ylabel("Price") plt.title("Stock Price Time Series") plt.show()
-
Day 3: Market Data Sources
- Objective: Explore different sources of financial market data.
- Topics: Data sources, APIs, and data providers.
-
Day 4: Accessing Market Data with Python
- Objective: Learn how to retrieve and use market data with Python libraries like yfinance or Alpha Vantage.
- Topics: API usage, data retrieval, and Python libraries for market data.
- Code Example: Accessing market data using yfinance.
- here is example from data in Bank Republik Indonesia
# Import necessary librariesimport yfinance as yf # For fetching stock datafrom sklearn.linear_model import LinearRegression # For creating a linear regression modelimport pandas as pd # For data manipulation and analysisimport numpy as np # For numerical operationsimport matplotlib.pyplot as plt # For data visualization
# Define the stock symbol and fetch historical datastock_symbol = 'BBRI.JK' # Example stock symbolstock = yf.Ticker(stock_symbol) # Creating a Ticker object for the stock symbolhistorical_data = stock.history(period="5y") # Fetching historical data for the past 5 years
# Considering only the 'Close' price for modelingdata = historical_data[['Close']] # Extracting 'Close' prices from the historical datadata.reset_index(level=0, inplace=True) # Resetting the index and converting date to a columndata.columns = ['Date', 'Price'] # Renaming columns for clarity
# Creating features (using only one feature for simplicity - the index)data['Index'] = np.arange(len(data)) # Adding an 'Index' column to the DataFrame
# Splitting the data into training and testing setssplit_index = int(0.8 * len(data)) # Determining the split index for training and testingtrain_data = data.iloc[:split_index] # Creating the training datatest_data = data.iloc[split_index:] # Creating the testing data
# Creating and fitting the linear regression modelmodel = LinearRegression() # Creating a Linear Regression modelmodel.fit(train_data[['Index']], train_data['Price']) # Fitting the model with training data
# Making predictions using the modeltest_data['Predictions'] = model.predict(test_data[['Index']]) # Making predictions for the test data
# Visualizing the predictions and actual valuesplt.figure(figsize=(12, 6)) # Creating a plot figureplt.title('Stock Price Prediction') # Setting the title of the plotplt.xlabel('Index') # Labeling the x-axisplt.ylabel('Stock Price') # Labeling the y-axisplt.plot(train_data['Date'], train_data['Price'], label='Training Data') # Plotting training dataplt.plot(test_data['Date'], test_data['Price'], label='Actual Stock Price') # Plotting actual stock pricesplt.plot(test_data['Date'], test_data['Predictions'], label='Predicted Stock Price', linestyle='dashed') # Plotting predicted stock pricesplt.legend() # Adding a legend to the plotplt.show() # Displaying the plot
import yfinance as yf # Define the stock symbol and date range stock_symbol = "AAPL" start_date = "2020-01-01" end_date = "2022-12-31" # Download historical data data = yf.download(stock_symbol, start=start_date, end=end_date)
- Day 5: Exercise
- Objective: Retrieve historical stock prices, analyze market data, and visualize price trends using Python.
Note: Week 3 focuses on time series analysis and accessing financial market data with Python.
3 months, 1 week ago
A reflection of using kanban flow and being minimalist
Recent newsToday is the consecutive day I want to use and be consistent with the Kanban flow! It seems it's perfect to limit my parallel and easily distractedness.
read more3 months, 2 weeks ago
3 months, 3 weeks ago
Podcast Bapak Dimas 2 - pindahan rumah
Recent newsVlog kali ini adalah terkait pindahan rumah!
read more3 months, 3 weeks ago
Podcast Bapak Dimas - Bapaknya Jozio dan Kaziu - ep 1
Recent newsSeperti yang saya cerita kan sebelumnya, berikut adalah catatan pribadi VLOG kita! Bapak Dimas
read more3 months, 3 weeks ago
Happy new year 2024 and thank you 2023!
Recent newsAs the new year starts, I want to revisit what has happened in 2023.
read more3 months, 3 weeks ago
Some notes about python and Zen of Python
Recent newsExplore Python syntax
Python is a flexible programming language used in a wide range of fields, including software development, machine learning, and data analysis. Python is one of the most popular programming languages for data professionals, so getting familiar with its fundamental syntax and semantics will be useful for your future career. In this reading, you will learn about Python’s syntax and semantics, as well as where to find resources to further your learning.
5 months ago
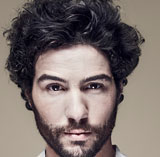
Collaboratively administrate empowered markets via plug-and-play networks. Dynamically procrastinate B2C users after installed base benefits. Dramatically visualize customer directed convergence without
Comments